Pandas DataFrame DataFrame.median() 函式
-
pandas.DataFrame.median()
語法 -
示例程式碼:
DataFrame.median()
方法沿列軸尋找中位數 -
示例程式碼:
DataFrame.median()
方法沿行軸尋找中位數 -
示例程式碼:
DataFrame.median()
方法忽略NaN
值來尋找中位數
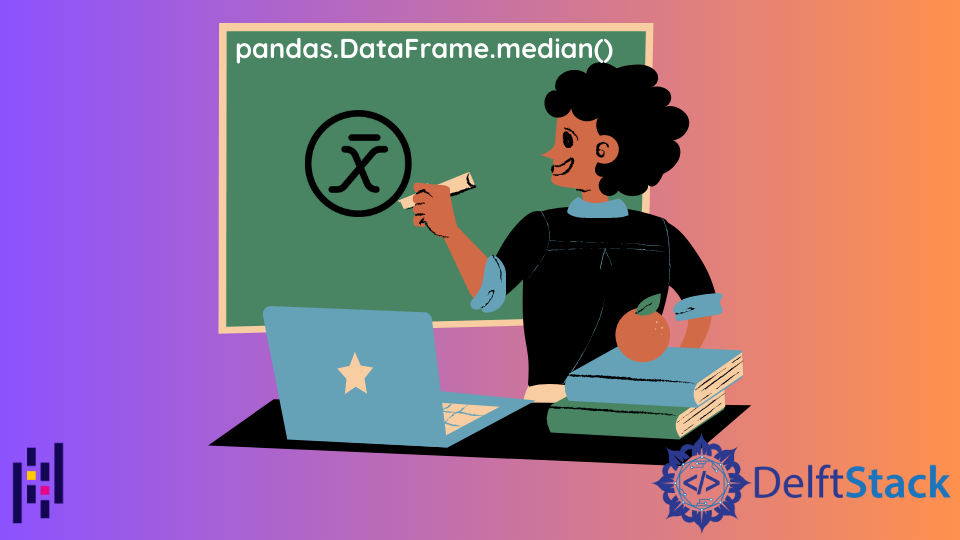
Python Pandas DataFrame.median()
函式計算 DataFrame 物件的元素沿指定軸的中位數。
中位數不是平均數,而是數字列表中數值的中間值。
pandas.DataFrame.median()
語法
pythonCopyDataFrame.median( axis=None,
skipna=None,
level=None,
numeric_only=None,
**kwargs)
引數
axis |
沿行(axis=0 )或列(axis=1 )找中位數 |
skipna |
布林型。排除 NaN 值(skipna=True )或包含 NaN 值(skipna=False ) |
level |
如果軸為 MultiIndex ,則沿特定級別尋找中位數 |
numeric_only |
布林型。布林型。對於 numeric_only=True ,只包括 float 、int 和 boolean 列 |
**kwargs |
函式的附加關鍵字引數 |
返回值
如果沒有指定 level
,則返回請求軸的中值的 Series
,否則返回中位數的 DataFrame
。
示例程式碼:DataFrame.median()
方法沿列軸尋找中位數
pythonCopyimport pandas as pd
df = pd.DataFrame({'X': [1, 2, 7, 5, 10],
'Y': [4, 3, 8, 2, 9]})
print("DataFrame:")
print(df)
medians=df.median()
print("medians of Each Column:")
print(medians)
輸出:
textCopyDataFrame:
X Y
0 1 4
1 2 3
2 7 8
3 5 2
4 10 9
medians of Each Column:
X 5.0
Y 4.0
dtype: float64
計算 X
和 Y
兩列的中位數,最後返回一個包含每列中位數的 Series
物件。
在 Pandas 中,要找到 DataFrame 中某一列的中位數,我們只呼叫該列的 median()
函式。
pythonCopyimport pandas as pd
df = pd.DataFrame({'X': [1, 2, 7, 5, 10],
'Y': [4, 3, 8, 2, 9]})
print("DataFrame:")
print(df)
medians=df["X"].median()
print("medians of Each Column:")
print(medians)
輸出:
pythonCopyDataFrame:
X Y
0 1 4
1 2 3
2 7 8
3 5 2
4 10 9
medians of Each Column:
5.0
它只給出 DataFrame
中的 X
列的中位數。
示例程式碼:DataFrame.median()
方法沿行軸尋找中位數
pythonCopyimport pandas as pd
df = pd.DataFrame({'X': [1, 2, 7, 5, 10],
'Y': [4, 3, 8, 2, 9],
'Z': [2, 7, 6, 10, 5]})
print("DataFrame:")
print(df)
medians=df.median(axis=1)
print("medians of Each Row:")
print(medians)
輸出:
textCopyDataFrame:
X Y Z
0 1 4 2
1 2 3 7
2 7 8 6
3 5 2 10
4 10 9 5
medians of Each Row:
0 2.0
1 3.0
2 7.0
3 5.0
4 9.0
dtype: float64
它計算所有行的中位數,最後返回一個包含每行中位數的 Series
物件。
在 Pandas 中,要想找到 DataFrame
中某一行的中位數,我們只呼叫 median()
函式來計算這一行的中位數。
pythonCopyimport pandas as pd
df = pd.DataFrame({'X': [1, 2, 7, 5, 10],
'Y': [4, 3, 8, 2, 9],
'Z': [2, 7, 6, 10, 5]})
print("DataFrame:")
print(df)
median=df.iloc[[0]].median(axis=1)
print("median of 1st Row:")
print(median)
輸出:
textCopyDataFrame:
X Y Z
0 1 4 2
1 2 3 7
2 7 8 6
3 5 2 10
4 10 9 5
median of 1st Row:
0 2.0
dtype: float64
它只給出了 DataFrame
中第一行數值的中位數。
我們使用 iloc
方法根據索引選擇行。
示例程式碼:DataFrame.median()
方法忽略 NaN
值來尋找中位數
我們使用 skipna
引數的預設值,即 skipna=True
,通過忽略 NaN
值,沿指定的軸找到 DataFrame
的中位數。
pythonCopyimport pandas as pd
df = pd.DataFrame({'X': [1, 2, 7, None, 10, 8],
'Y': [None, 3, 8, 2, 9, 6],
'Z': [2, 7, 6, 10, None, 5]})
print("DataFrame:")
print(df)
median=df.median(skipna=True)
print("medians of Each Row:")
print(median)
輸出:
textCopyDataFrame:
X Y Z
0 1.0 NaN 2.0
1 2.0 3.0 7.0
2 7.0 8.0 6.0
3 NaN 2.0 10.0
4 10.0 9.0 NaN
5 8.0 6.0 5.0
medians of Each Row:
X 7.0
Y 6.0
Z 6.0
dtype: float64
如果我們設定 skipna=True
,它會忽略 DataFrame 中的 NaN
。它允許我們通過忽略 NaN
值來計算 DataFrame
沿列軸的中位數。
pythonCopyimport pandas as pd
df = pd.DataFrame({'X': [1, 2, 7, None, 10],
'Y': [5, 3, 8, 2, 9],
'Z': [2, 7, 6, 10, 4]})
print("DataFrame:")
print(df)
median=df.median(skipna=False)
print("medians of Each Row:")
print(median)
輸出:
textCopyDataFrame:
X Y Z
0 1.0 5 2
1 2.0 3 7
2 7.0 8 6
3 NaN 2 10
4 10.0 9 4
medians of Each Row:
X NaN
Y 5.0
Z 6.0
dtype: float64
這裡,我們得到的是 NaN
值作為 X
列的中位數,因為 X
列中存在 NaN
值。
Founder of DelftStack.com. Jinku has worked in the robotics and automotive industries for over 8 years. He sharpened his coding skills when he needed to do the automatic testing, data collection from remote servers and report creation from the endurance test. He is from an electrical/electronics engineering background but has expanded his interest to embedded electronics, embedded programming and front-/back-end programming.
LinkedIn